
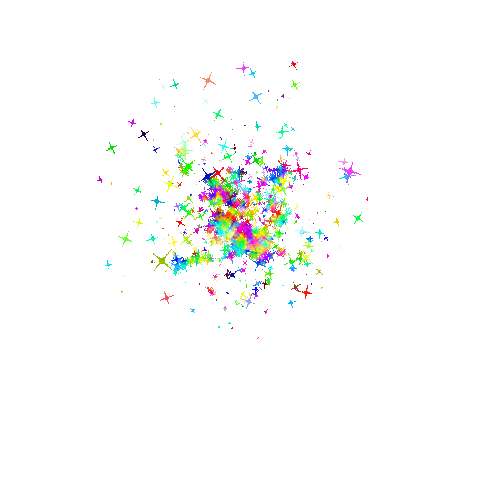





ESTD 2008
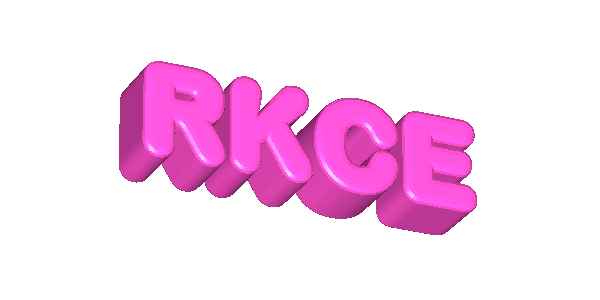


Kethanakonda (V), Ibrahimpatnam (M), Vijayawada, AMARAVATI - 521456
COUNSELLING CODE
(Approved by AICTE, New Delhi & Affiliated to JNTUK, Kakinada & SBTET, Amaravati )
Department of Computer Science & Engineering (Data Science)

Time Tables
Materials
Data science can be defined as a blend of mathematics, business acumen, tools, algorithms and machine learning techniques, all of which help us in finding out the hidden insights or patterns from raw data which can be of major use in the formation of big business decisions.
In data science, one deals with both structured and unstructured data. The algorithms also involve predictive analytics in them. Thus, data science is all about the present and future. That is, finding out the trends based on historical data which can be useful for present decisions and finding patterns which can be modelled and can be used for predictions to see what things may look like in the future.
Data Science is an amalgamation of Statistics, Tools and Business knowledge. So, it becomes imperative for a Data Scientist to have good knowledge and understanding of these.
Why To Learn Data Science?
With the amount of data that is being generated and the evolution in the field of Analytics, Data Science has turned out to be a necessity for companies. To make most out of their data, companies from all domains, be it Finance, Marketing, Retail, IT or Bank. All are looking for Data Scientists. This has led to a huge demand for Data Scientists all over the globe. With the kind of salary that a company has to offer and IBM is declaring it as trending job of 21st century, it is a lucrative job for many. This field is such that anyone from any background can make a career as a Data Scientist.
Components Of Data Science
Data Science consists of 3 parts namely:
Machine Learning: Machine Learning involves algorithms and mathematical models, chiefly employed to make machines learn and prepare them to adapt to everyday advancements. For example, these days, time series forecasting is very much in use in trading and financial systems. In this, based on historical data patterns, the machine can predict the outcomes for the future months or years. This is an application of machine learning.
Big Data: Everyday, humans are producing so much of data in the form of clicks, orders, videos, images, comments, articles, RSS Feeds etc. These data are generally unstructured and is often called as Big Data. Big Data tools and techniques mainly help in converting this unstructured data into a structured form. For example, suppose someone wants to track the prices of different products on e-commerce sites. He/she can access the data of the same products from different websites using Web APIs and RSS Feeds. Then convert them into structured form.
Business Intelligence: Each business has and produces too much data every day. This data when analysed carefully and then presented in visual reports involving graphs, can bring good decision making to life. This can help the management in taking the best decision after carefully delving into patterns and details the reports bring to life.
Skills Required
Skills required to become a data scientist include:
Tools
-
In-depth knowledge in R: R is used for data analysis, as a programming language, as an environment for statistical analysis, data visualization
-
Python coding: Python is majorly preferred to implement mathematical models and concepts because python has rich libraries/packages to build and deploy models.
-
MS Excel: Microsoft Excel is considered a basic requirement for all data entry jobs. It is of great use in data analysis, applying formulae, equations, diagrams out of a messy lot of data.
-
Hadoop Platform: It is an open source distributed processing framework. It is used for managing the processing and storage of big data applications.
-
SQL database/coding: It is mainly used for the preparation and extraction of datasets. It can also be used for problems like Graph and Network Analysis, Search behaviour, fraud detection etc.
-
Technology: Since there is so much unstructured data out there, one also should know how to access that data. This can be done in a variety of ways, via APIs, or via web servers.
Techniques
-
Mathematical Expertise: Data scientists also work on machine learning algorithms such as regression, clustering, time series etc which require a very high amount of mathematical knowledge since they themselves are based on mathematical algorithms.
-
Working with unstructured data: Since most of the data produced every day, in the form of images, comments, tweets, search history etc is unstructured, it is a very useful skill in today’s market to know how to convert this unstructured into a structured form and then working with them.
​